
Read This On Our Main Website: https://psoec.com/use-machine-learning-in-the-evolving-landscape-of-real-data/?feed_id=2192&_unique_id=61b1ee8979c03
[ad_1]
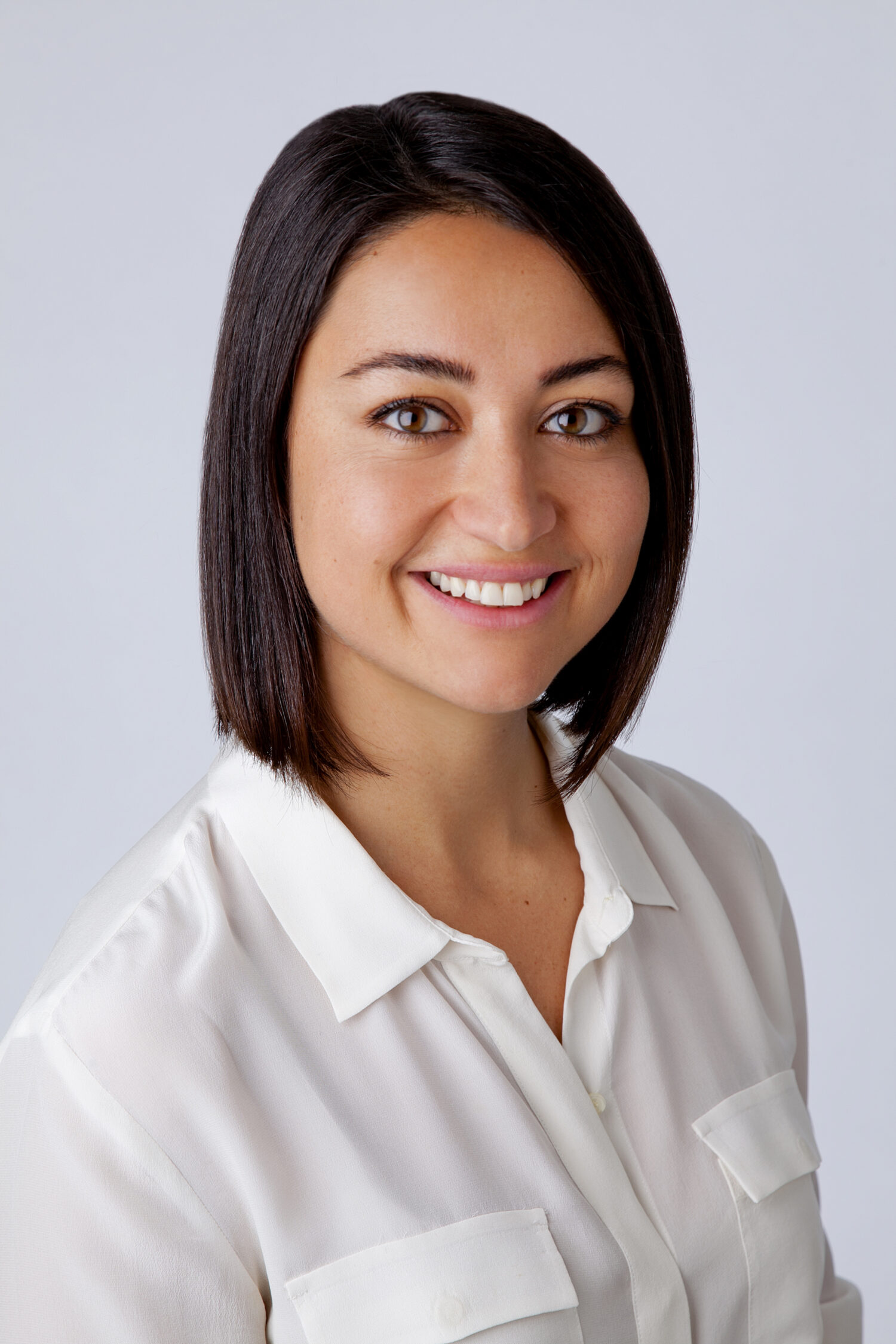
According to the Food and Drug Administration (FDA.)), Real-World-Data (RWD) refers to routinely collected knowledge associated to affected person well being and well being care supply, and Real-World-Evidence (RWE) is scientific proof of use and potential advantages or dangers of a medical gadget derived from the evaluation by RWD. Both RWD and RWE have been attracting growing consideration within the healthcare industry for years, and rightly so, contemplating that the healthcare analytics market is predicted to extend by 1 average annual growth rate of 28.9% between now and 2026. There is little doubt that there's innumerable insights on this huge treasure trove of information that would optimize medical care, assist medical doctors diagnose illnesses sooner, and enhance remedy methods - if solely we may determine them.
This knowledge revolution that we're witnessing within the healthcare industry requires the suitable instruments and approaches to work with increased dimensional knowledge sources in an effort to actually extract the insights buried in RWD. Machine studying, an space of synthetic intelligence (AI) made up of a group of methodologies that target algorithmic studying of environment friendly knowledge representations and extracting insights from knowledge, holds nice promise and has continued on within the context of RWD within the industry Gained significance. In reality, in January 2021, the FDA approved a synthetic intelligence / machine studying (AI / ML) primarily based motion plan for software program as a medical gadget (SaMD), a transparent indication of the explosive progress and legitimacy of know-how in healthcare. In 2018, the Centers for Medicare and Medicaid Services launched their first AI health outcomes challenge, a cross-industry competitors to innovate how AI could be carried out in present and future well being fashions.
Healthcare analytics is at a tipping level in adoption, and it's important that call makers develop clear methods for deploying ML-driven applied sciences. The actual query just isn't why Companies ought to use machine studying, nevertheless how. How do corporations use these superior strategies in an space like healthcare the place there's an abundance of ambiguity and typically intuitive decision-making? When are ML approaches preferable and when do they provide benefits over standard statistical approaches? The reply is determined by the sort and quantity of information out there, in addition to the analysis targets. It's additionally vital to know the kinds of use instances and analytics that ML can shine in and the way these functions can advance the industry.
The two cultures: machine studying and statistics
ML affords a number of advantages that well being analytics can enhance for initiatives comparable to product improvement and introduction, understanding affected person populations, figuring out unmet medical wants, predicting affected person outcomes or illness recurrence, and verifying real-world drug efficiency. ML strategies work finest with giant, clinically wealthy knowledge units, the place the relationships between knowledge objects could be very nonlinear and complicated. As the variety of knowledge parts and the complexity of the associations between them enhance, conventional approaches that require inflexible assumptions concerning the knowledge, not like ML approaches that be taught the patterns within the knowledge and make little or no assumptions concerning the knowledge era processes, lose a poorer efficiency.
Traditional statistical approaches have lengthy centered on inference, whereas ML's principal focus has been on prediction as a result of it might probably be taught generalizable patterns in knowledge. ML strategies can even work with out a priori hypotheses - one primarily based on assumed ideas and conclusions from earlier analysis - and might counsel new insights that may in any other case be ignored in advanced knowledge units. The primary reality is that the predictive efficiency of ML fashions will increase on the expense of mannequin interpretability. For instance, subtle neural community fashions can often outperform different approaches, however additionally they require extra effort to clarify and interpret. The key questions then turn out to be what sort of inference is the principle aim and whether or not predictive efficiency or interpretability is extra vital.
In abstract, the principle benefits of ML approaches are their skill to work with out a priori speculation, which permits for max profit from giant quantities of information and in the end the power to suggest new insights and supply higher management of mix-ups. In addition, ML permits environment friendly dealing with of high-dimensional knowledge units and modeling of nonlinear phenomena and interactions, which may result in higher prediction efficiency and mannequin generalization. It can be vital to emphasise that, regardless of the benefits, ML strategies should not essentially an alternative choice to conventional statistical approaches, however slightly should be used to enrich, increase and reinforce information gained utilizing conventional strategies. ML approaches are a beneficial addition to the RWE toolbox and, in the proper context, assist obtain the true worth of RWD.
A use case for machine studying in predicting affected person outcomes
One method to higher perceive how machine studying can enhance well being analytics is to look at a use case the place the know-how has proven promising outcomes. For instance, we just lately labored on a examine aimed toward figuring out predictors of inpatient relapse among the many administrative entitlement knowledge of sufferers with a number of sclerosis (MS), and created a data-driven resolution rule to differentiate between inpatients with and with out inpatient relapse to differentiate. There are almost a million folks dwelling with MS within the United States, and relapse is often related to incapacity development and worsening outcomes. Understanding what causes relapse can result in higher illness administration and thrust back extra severe results. To decide this, we used ML approaches that target maximizing the insights from RWD to develop strong, sturdy predictive fashions whereas characteristic choice is in progress.
Using machine studying fashions, we achieved an space beneath the curve (AUC) of 79.3%, a sensitivity of 69% and a specificity of 75%. We recognized the first predictors of MS relapse, together with a earlier inpatient or emergency room go to with a prognosis of MS, the variety of MS-related encounters, the variety of comorbidities, using residence care companies, and long-life medical units , Epilepsy or convulsions, paralysis, urinary tract infections and using muscle relaxants, anticonvulsants and antidepressants. Notable components defending in opposition to relapse have been elevated PDC, older age, feminine gender, and disease-modifying therapies given by infusion. We have been additionally capable of outline a compact resolution rule that states that sufferers usually tend to have a relapse in the event that they:
- Have 30 or extra distinctive comorbidities, or
- Have a earlier go to to the emergency room with a prognosis of MS and 10 or extra earlier MS-related encounters, or
- Have 20 or extra earlier MS-related encounters.
The benefit of this resolution rule is that it's fully data-driven and evidence-based. It's very interpretable and intuitive. After all, it makes use of key figures which might be out there in all claims knowledge units and might due to this fact be transferred to different databases. After acceptable exterior validation, this resolution rule can be utilized as a proxy for the severity of the illness and used to stratify sufferers with regard to their chance of relapse in database research. It will also be used to streamline intervention administration to proactively stop potential relapses by figuring out sufferers who're prone to get probably the most profit from further preventive interventions to extend remedy adherence or a brand new remedy routine. These proactive interventions can enhance their high quality of life and cut back the necessity for extra interactions with the well being system, which may reduce strain on already overburdened service supplier organizations.
The subsequent frontier for machine studying
ML approaches have the uncanny skill to sift by means of high-dimensional, real-world knowledge units and filter out key insights that may result in healthcare value financial savings and higher high quality of life for sufferers. The sciences of AI and ML are advancing at a quick tempo, and new applied sciences and platforms permit science to be utilized to actual questions, however the breakthrough for the profitable adoption of those strategies is not only achieved by means of elevated computing energy, extra knowledge, or higher software program , but in addition by means of a deep understanding of downside sorts that may finest be formulated as ML issues, in addition to by means of instruments and strategies that make the fashions clear and explainable.
Companies seeking to implement ML of their analytics packages can profit from a mature, health-specific platform that helps them overcome the anomaly and complexity of well being knowledge. As extra corporations start to embrace the know-how and learn to correctly use its capabilities, it has the potential to remodel the healthcare industry and the way knowledge and analytics will probably be used sooner or later.
About Zeynep Icten, Ph.D.
Zeynep is Director of Data Science Solutions at Panalgo the place she oversees the coaching and assist of the IHD Data Science module. Her experience lies in data-driven approaches in well being evaluation utilizing actual knowledge sources. Zeynep has a wealth of expertise working with numerous key healthcare gamers together with pharmaceutical corporations, payers and suppliers, serving to them use knowledge science and machine studying strategies to generate actionable insights. She has an MS and Ph.D. in industrial engineering from the University of Pittsburgh.
[ad_2]
Read This On Our Main Website: https://psoec.com/use-machine-learning-in-the-evolving-landscape-of-real-data/?feed_id=2192&_unique_id=61b1ee8979c03
Comments
Post a Comment